ACT-America: Ensembles of Biogenic C Fluxes for North America, 2003-2018
Download this document in PDF
Data Set Version: V1.1 (updated on July 12, 2019)
Note: In July 2019, the 2018 results were added and 2017 simulation were rerun with updated meteorological driver dataset.
Summary
This data set provides gridded, model-derived gross
primary productivity (GPP), ecosystem respiration (RECO), and net ecosystem
exchange (NEE) of CO2 biogenic fluxes and their uncertainties at
monthly and 3-hourly time scales over 2003-2018 on a 463-m resolution grid for
the conterminous United States (CONUS) and also on a 5-km resolution grid for
North America (NA). The 5-km results are further upscaled to a half-degree resolution. The biogeochemical model is Carnegie Ames Stanford Approach
(CASA).
There are 708 files in NetCDF v4 format with this data set. This includes 420 files containing ensemble members of each carbon flux and 288 files that are the mean and standard deviation across ensemble members.
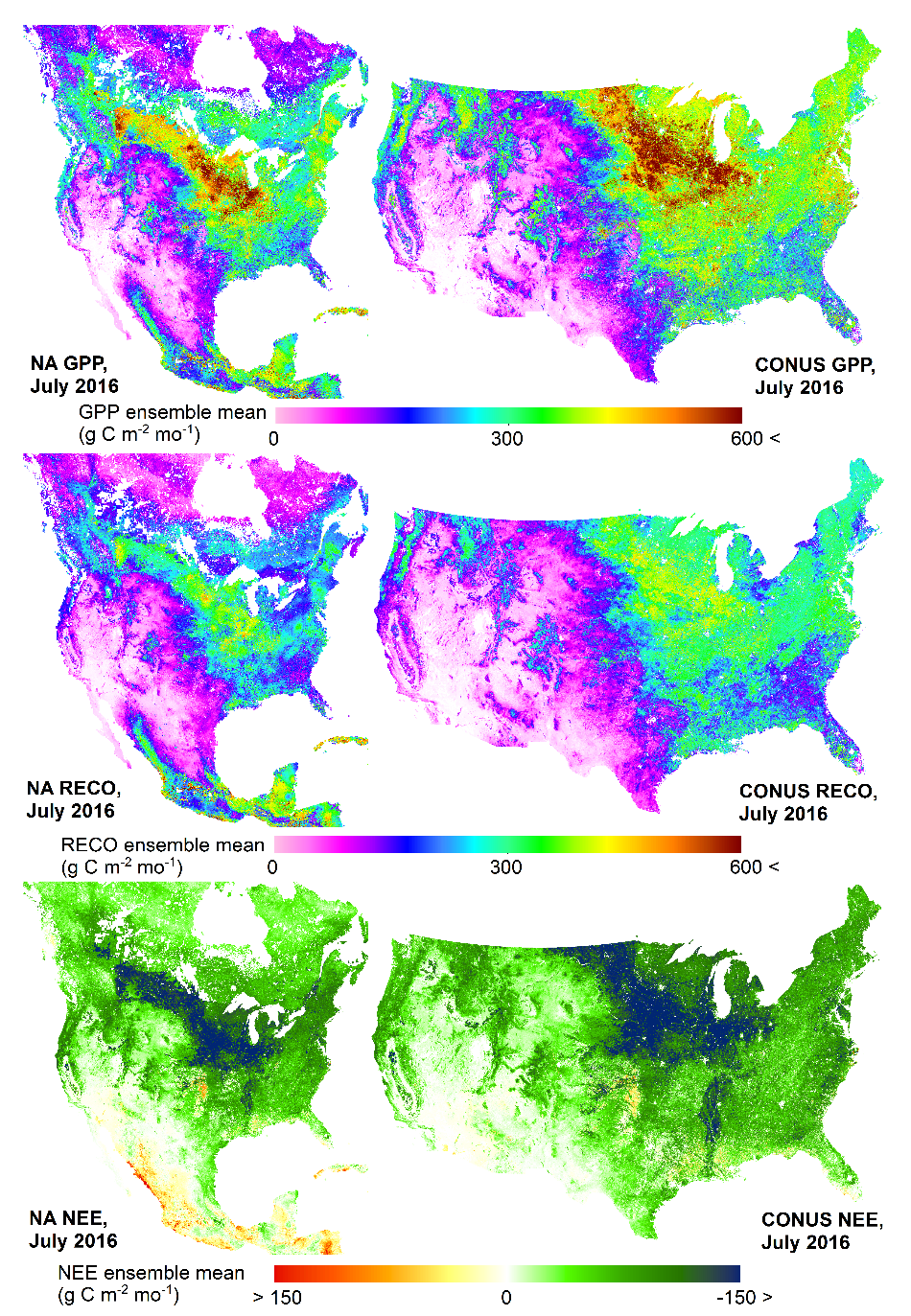
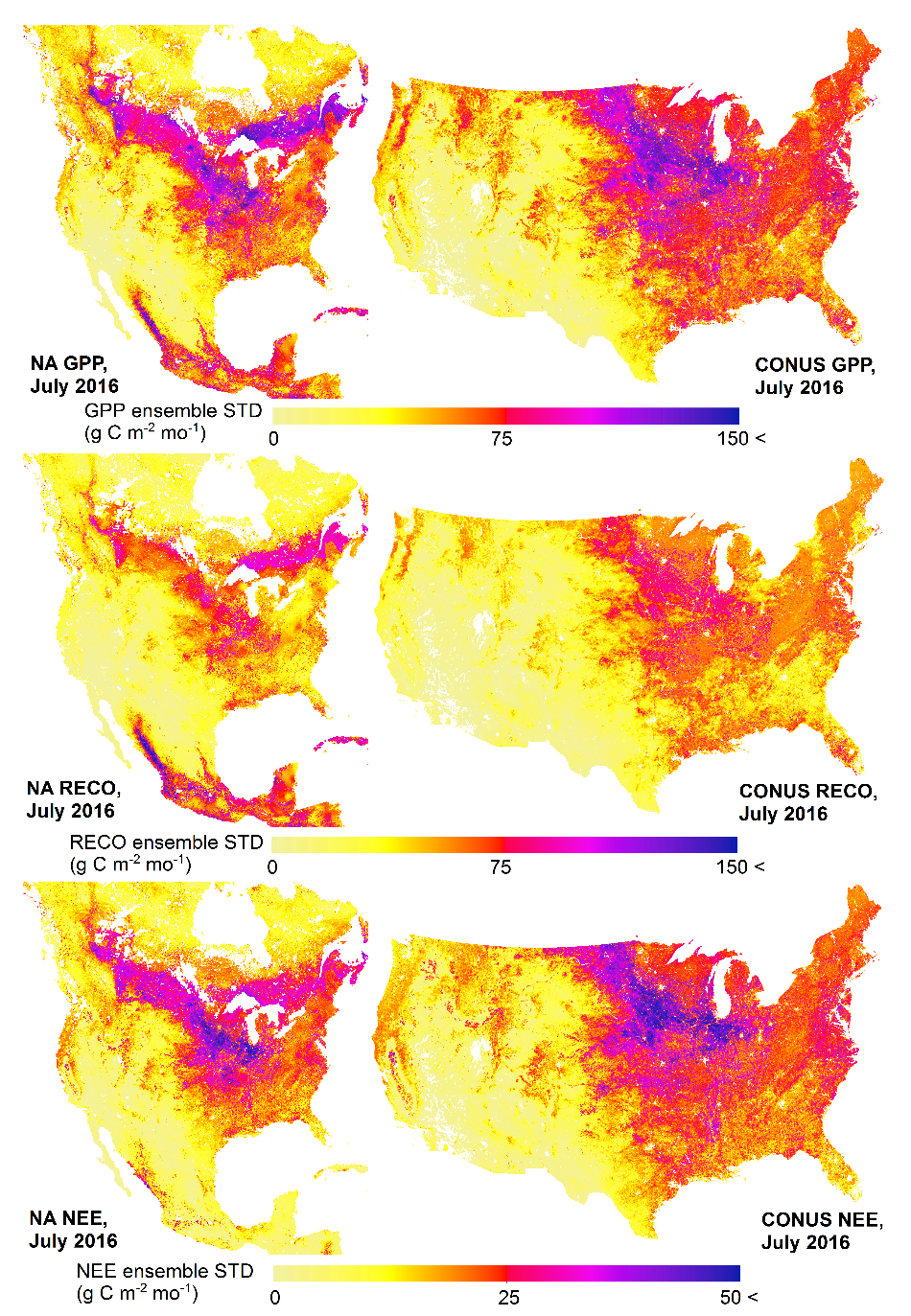
Figure 1. Mean and standard deviation of CASA L2
ensembles for three carbon fluxes (GPP, RECO, and NEE) and at 463-m resolution
for the conterminous US (CONUS) and at 5-km resolution for North America (NA)
in July of 2016.
Citation
Yu Zhou, Christopher A. Williams, Thomas Lauvaux, Sha
Feng, Ian Baker, Yaxing Wei, Scott Denning, Klaus Keller, Kenneth J. Davis.
ACT-America: Gridded Ensembles of Surface Biogenic Carbon Fluxes for North
America and the Conterminous United States, 2003-2018. ORNL DAAC, Oak Ridge,
Tennessee, USA. https://doi.org/10.3334/ORNLDAAC/1675.
Table of
Contents
1. Data Set Overview
2. Data Characteristics
3. Application and Derivation
4. Quality Assessment
5. Data Acquisition, Materials, and Methods
6. Data Access
7. References
1.
Data Set Overview
This dataset
that contains the second-level (L2) ensemble member estimates of surface
biogenic CO2 exchanges between land and atmosphere across portions
of North America, and including three carbon fluxes: gross primary productivity (GPP), ecosystem
respiration (RECO), and net ecosystem exchange (NEE). Carbon flux
ensembles are derived from Carnegie
Ames Stanford Approach (CASA) biogeochemical model (Potter et al. 1993; Randerson et al. 1996) with 27
perturbed parameter sets. This product contains carbon fluxes for two spatial
domains, the conterminous United States and North America and at two temporal
scales, monthly and 3-hourly.
Project: Atmospheric Carbon and Transport (ACT-America)
The
ACT-America, or Atmospheric Carbon and Transport - America, project is a NASA
Earth Venture Suborbital-2 mission to study the transport and fluxes of
atmospheric carbon dioxide and methane across three regions in the eastern
United States. Each flight campaign will measure how weather systems transport
these greenhouse gases. Ground-based measurements of greenhouse gases were
also-collected. Better estimates of greenhouse gas sources and sinks are needed
for climate management and for prediction of future climate.
Spatial Coverage: Conterminous United States and North
America
Spatial Resolution: 463m, 5km, and half degree
Temporal Coverage: 2003-01-01 to 2018-12-31
Temporal Resolution: Monthly and 3-hourly (3-hourly data
is available for North America domain in 2016-2018; other temporal and
spatial spans can be generated at user’s end with provided R script)
Site boundaries: (All latitudes and longitudes are
given in decimal degrees)
Site |
Westernmost Longitude |
Easternmost Longitude |
Northernmost Latitude |
Southernmost Latitude |
CONUS |
-130.1748 |
-60.5999 |
55.3236 |
20.0276 |
NA |
-175.5350 |
-24.7704 |
70.3800 |
0.7843 |
Data Description:
There are 708 files in netCDF v4 format in this data set, including 420 files (204 monthly and 216 3-hourly files) containing ensemble members of each carbon flux and 288 files are the mean and standard deviation across ensemble members. CONUS (conterminous United States) files are at 463m×463m spatial resolution, NA (North America) files are at 5-km×5-km resolution, and NA_HalfDeg (upscaled North America) files are at half-degree resolution. The time dimension is defined as the middle time point of each time period (e.g., 15th day of Marches for monthly files; 1.5 hours of the first three-hour for 3-hourly files). Fill value and missing values are -9999 for all files.
Data file naming convention:
CASAensemble_CASA_LEVEL_Ensemble_TIMESCALE_Biogenic_CARBONFLUX_SPATIALDOMAIN_YEAR(MONTH).nc4
CASA_LEVEL_Ensemble_STATISTIC_TIMESCALE_Biogenic_CARBONFLUX_SPATIALDOMAIN_YEAR(MONTH).nc4
Where
CASALEVEL is the level of data product, we currently provide Level-2 (L2) and Level-2B (L2B).
TIMESCALE is either monthly or 3-hourly.
STATISTIC is the mean or standard deviation (STD) across
ensemble members.
CARBONFLUX is GPP, RECO or NEE.
SPATIALDOMAIN is CONUS, NA, or NA_HalfDeg.
YEAR is the year of simulation.
MONTH is simulated month, which only used for 3-hourly data
Example file names:
CASA_L2B_Ensemble_Monthly_Biogenic_GPP_NA_2005.nc4
CASA_L2_Ensemble_Mean_Monthly_Biogenic_NEE_CONUS_2004.nc4
CASA_L2_Ensemble_3-Hourly_Biogenic_RECO_NA_201605.nc4
Spatial Reference Properties:
North America Data
Projection:
Lambert Conformal Conic 2SP
Parameters:
projection units: meters
datum (spheroid): GCS_unnamed_ellipse (from NARR data)
Semi major
Axis: 6371200.0
Semi minor Axis: 6371200.0
Inverse Flattening: 0.0
1st standard parallel: 50 deg N
2nd standard parallel: 50 deg N
Central meridian: -107deg (W)
latitude of origin: 50 deg N
false easting: 0
false northing: 0
Upscaled North America Data
Projection: WGS 1984
Parameters:
Angular Unit: Degree (0.0174532925199433)
Prime Meridian: Greenwich (0.0)
Datum: D_WGS_1984
Semi major Axis: 6378137.0
Semi minor Axis: 6356752.314245179
Inverse Flattening: 298.257223563
Conterminous United States Data
Projection:
Lambert Conformal Conic
Parameters:
projection units: meters
datum (spheroid): GRS_1980
Semi major
Axis: 6378137.0
Semi minor Axis:
6356752.314140356
Inverse Flattening:
298.257222101
1st standard parallel: 50 deg N
2nd standard parallel: 50 deg N
Central meridian: -107deg (W)
Latitude of origin: 50 deg N
false easting: 0
false northing: 0
3-Hourly NARR files:
These files are
examples of ancillary data from 3-hourly NARR data set (https://rda.ucar.edu/datasets/ds608.0/index.html#!description)
to use the R script for temporal downscaling.
NARR_YEARMONTH_3h_FACTOR.tif
Where
YEAR is the year for temporal downscaling.
MONTH is selected month, which only used for 3-hourly data
FACTOR is either dwsw (downward shortwave radiation) or airt
(air temperature at 2-m height).
Our product has finer spatial resolutions and a
relatively long time span comparing to other available product. It could be
used to access surface biogenic carbon fluxes across multiple spatial (hundred
meters to continental) and temporal (hourly to annual) scales can give an
indication of carbon cycle processes under different weather patterns and
feedbacks to climate change.
Our ensemble product provides not only carbon flux
estimates but also the uncertainty range. This data product also could serve as
prior
surface biogenic carbon fluxes for atmospheric inversion studies.
To test and confirm the accuracy of our monthly
ensemble, the assessment was evaluated by a set of ground-truth data of
measured carbon fluxes from the AmeriFlux database (sites are listed in Table 1)
and other carbon flux products including 3-hourly MsTMIP modeled ensemble (Huntzinger et al. 2013;
Fisher et al. 2016; Huntzinger et al. 2016), CarbonTracker 2017 (CT2017, Peters et al. 2007), SiB3 (Baker et al. 2008;
Baker et al. 2013) from 2006 to 2010.
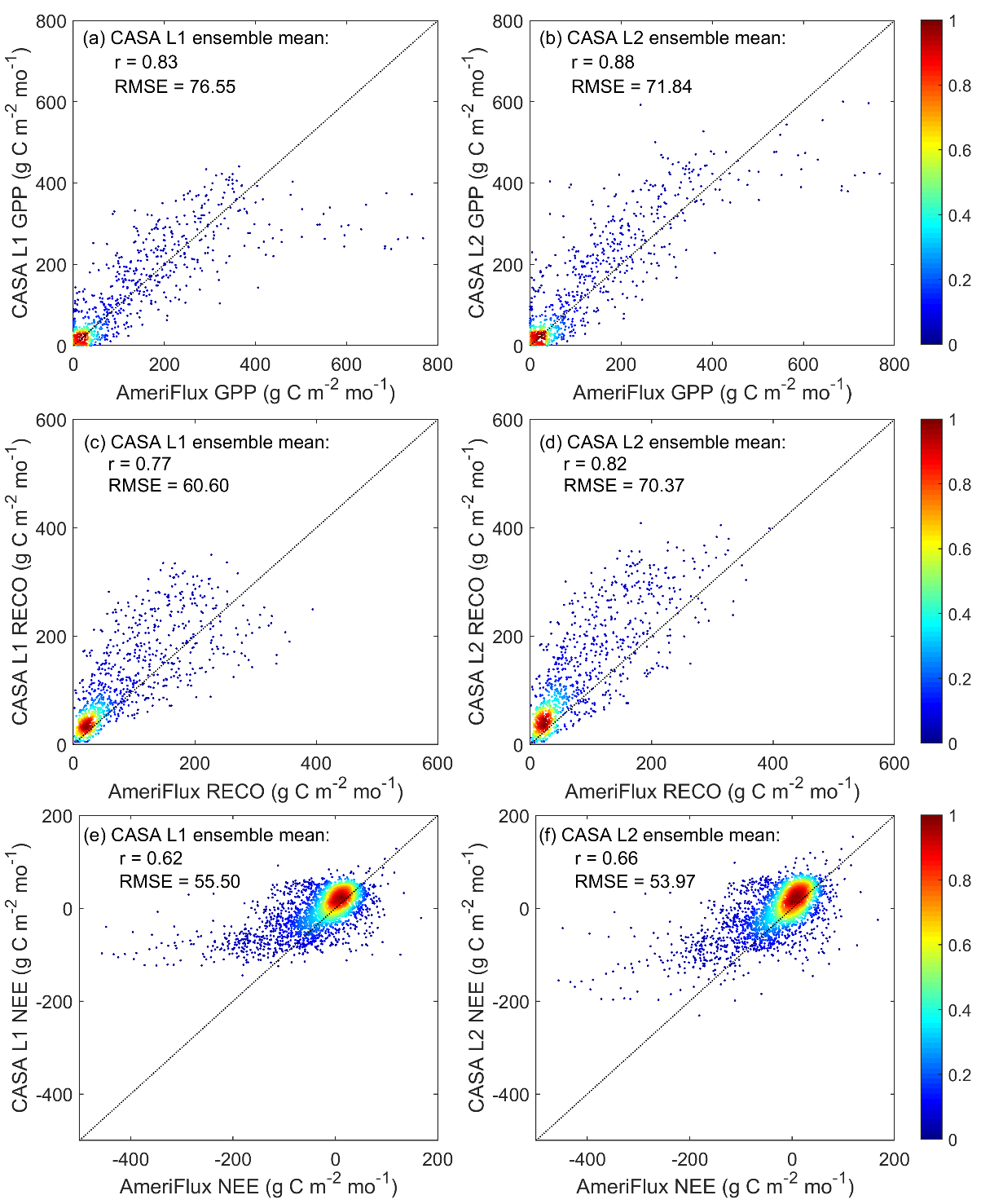
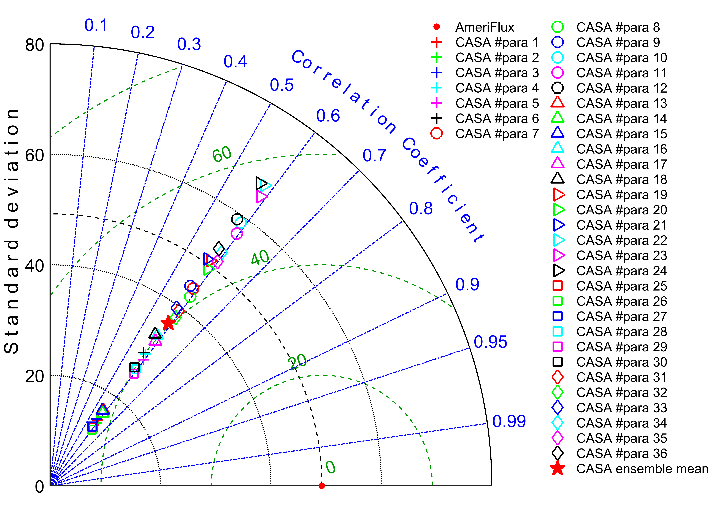
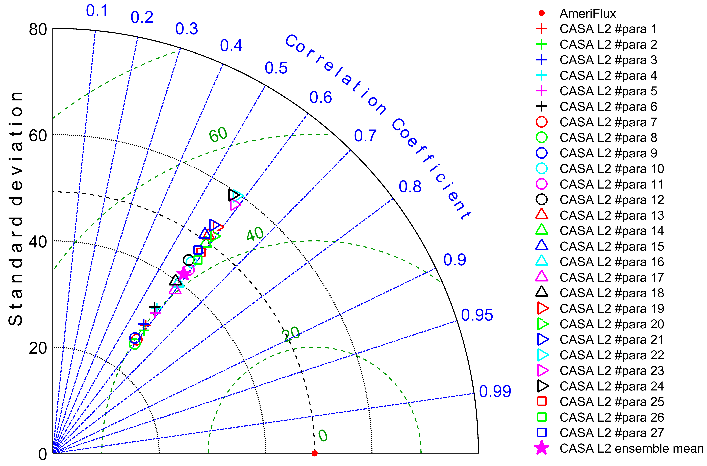
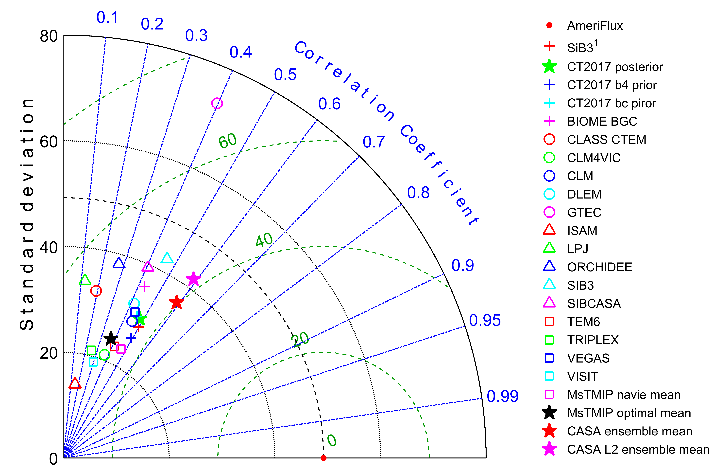
Table 1.
List of AmeriFlux tower sites used in the quality assessment.
Site ID |
Start Year |
End Year |
Lat |
Lon |
IGBP |
Reference |
US-AR1 |
2009 |
2012 |
36.4 |
-99.4 |
GRA |
Billesbach
et al. 2016a |
US-AR2 |
2009 |
2012 |
36.6 |
-99.6 |
GRA |
Billesbach
et al. 2016b |
US-ARb |
2005 |
2006 |
35.5 |
-98.0 |
GRA |
Torn
2006a |
US-ARc |
2005 |
2006 |
35.5 |
-98.0 |
GRA |
Torn
2006b |
US-ARM |
2003 |
2012 |
36.6 |
-97.5 |
CRO |
Fischer
et al. 2007 |
US-Blo |
1997 |
2007 |
38.9 |
-120.6 |
ENF |
Goldstein
et al. 2000 |
US-Cop |
2001 |
2007 |
38.1 |
-109.4 |
GRA |
Bowling
2007 |
US-EML |
2008 |
63.9 |
-149.3 |
OSH |
Belshe et
al. 2012 |
|
US-GBT |
1991 |
2006 |
41.4 |
-106.2 |
ENF |
Massman
2006 |
US-GLE |
2004 |
2014 |
41.4 |
-106.2 |
ENF |
Frank et
al. 2014 |
US-Goo |
2002 |
2006 |
34.3 |
-89.9 |
GRA |
Wilson
and Meyers 2007 |
US-Ha1 |
1991 |
2012 |
42.5 |
-72.2 |
DBF |
Urbanski
et al. 2007 |
US-Ho2 |
1999 |
45.2 |
-68.7 |
ENF |
Hollinger
et al. 1999 |
|
US-Ho3 |
2000 |
45.2 |
-68.7 |
ENF |
Hollinger
et al. 1999 |
|
US-IB2 |
2004 |
2011 |
41.8 |
-88.2 |
GRA |
Matamala
2018 |
US-KFS |
2007 |
39.1 |
-95.2 |
GRA |
Brunsell
2018a |
|
US-Kon |
2006 |
39.1 |
-96.6 |
GRA |
Brunsell
2018b |
|
US-KS2 |
2003 |
2006 |
28.6 |
-80.7 |
CSH |
Powell et
al. 2006 |
US-Lin |
2009 |
2010 |
36.4 |
-119.8 |
CRO |
Fares
2010 |
US-LPH |
2002 |
42.5 |
-72.2 |
DBF |
Hadley
2018 |
|
US-Me2 |
2002 |
2014 |
44.5 |
-121.6 |
ENF |
Thomas et
al. 2009 |
US-Me3 |
2004 |
2009 |
44.3 |
-121.6 |
ENF |
Vickers
et al. 2009 |
US-Me6 |
2010 |
44.3 |
-121.6 |
ENF |
Ruehr et
al. 2012 |
|
US-MMS |
1999 |
39.3 |
-86.4 |
DBF |
Schmid et
al. 2000 |
|
US-Mpj |
2007 |
34.4 |
-106.2 |
OSH |
Litvak
2018a |
|
US-MRf |
2005 |
44.6 |
-123.6 |
ENF |
Law 2018 |
|
US-Ne1 |
2001 |
41.2 |
-96.5 |
CRO |
Verma et
al. 2005 |
|
US-Ne2 |
2001 |
41.2 |
-96.5 |
CRO |
Verma et
al. 2005 |
|
US-Ne3 |
2001 |
41.2 |
-96.4 |
CRO |
Verma et
al. 2005 |
|
US-NR1 |
1998 |
40.0 |
-105.5 |
ENF |
Monson et
al. 2002 |
|
US-Oho |
2004 |
2013 |
41.6 |
-83.8 |
DBF |
Noormets
et al. 2008 |
US-PFa |
1995 |
45.9 |
-90.3 |
MF |
Desai et
al. 2015 |
|
US-Prr |
2010 |
2014 |
65.1 |
-147.5 |
ENF |
Nakai et
al. 2013 |
US-Ro2 |
2003 |
2018 |
44.7 |
-93.1 |
CRO |
Baker and
Griffis 2017 |
US-SRC |
2008 |
2014 |
31.9 |
-110.8 |
OSH |
Kurc 2018 |
US-SRG |
2008 |
2014 |
31.8 |
-110.8 |
GRA |
Scott et
al. 2015 |
US-SRM |
2004 |
2014 |
31.8 |
-110.9 |
WSA |
Scott et
al. 2009 |
US-Sta |
2005 |
2009 |
41.4 |
-106.8 |
OSH |
Ewers and
Pendall 2009 |
US-Syv |
2001 |
46.2 |
-89.3 |
MF |
Desai et
al. 2005 |
|
US-Ton |
2001 |
38.4 |
-121.0 |
WSA |
Fischer
et al. 2007 |
|
US-Twt |
2009 |
2017 |
38.1 |
-121.7 |
CRO |
Hatala et
al. 2012 |
US-UMB |
2000 |
45.6 |
-84.7 |
DBF |
Gough et
al. 2008 |
|
US-UMd |
2007 |
45.6 |
-84.7 |
DBF |
Gough et
al. 2018 |
|
US-Var |
2000 |
38.4 |
-121.0 |
GRA |
Fischer
et al. 2007 |
|
US-WCr |
1999 |
45.8 |
-90.1 |
DBF |
Cook et
al. 2004 |
|
US-Whs |
2007 |
31.7 |
-110.1 |
OSH |
Scott et
al. 2015 |
|
US-Wi1 |
2003 |
2003 |
46.7 |
-91.2 |
DBF |
Chen
2003a |
US-Wi2 |
2003 |
2003 |
46.7 |
-91.2 |
ENF |
Chen
2003b |
US-Wi3 |
2002 |
2004 |
46.6 |
-91.1 |
DBF |
Chen
2005a |
US-Wi5 |
2004 |
2004 |
46.7 |
-91.1 |
ENF |
Chen 2004 |
US-Wi6 |
2002 |
2003 |
46.6 |
-91.3 |
OSH |
Chen
2003c |
US-Wi7 |
2005 |
2005 |
46.6 |
-91.1 |
OSH |
Chen
2005a |
US-Wi9 |
2004 |
2005 |
46.6 |
-91.1 |
ENF |
Chen
2005b |
US-Wjs |
2007 |
34.4 |
-105.9 |
OSH |
Litvak
2018b |
|
US-Wkg |
2004 |
2014 |
31.7 |
-109.9 |
GRA |
Scott et
al. 2010 |
5. Data Acquisition, Materials, and Methods
5.1 CASA description
The modeling
approach is based on the CASA biogeochemical model
(Potter et al. 1993; Randerson et al. 1996). In CASA, NPP is calculated with a light use efficiency model driven by the absorbed fraction of photosynthetically active
radiation (fPAR) and scaled by
maximum light use efficiency (Emax),
temperature scalar (TNPP)
and moisture stresses (WNPP)
at
spatial location (x, y) and time (t) (Eq. 1). WNPP is derived based on a
ratio of estimated evapotranspiration to potential evapotranspiration, varying
from 0.5 in arid ecosystem to 1 in very wet ecosystem. TNPP is defined as T1×T2low×T2high. T1 reflects
the empirical observation that plants in very cold habitats typically have low
maximum growth rate (Eq. 2). T2
reflects the concept that the efficiency of light utilization should be
depressed when plants are growing at temperatures displaces from their optimum
(Eq. 3 and 4). T2 has an
asymmetric bell shape that falls off more quickly at high than at low
temperatures. Topt is
defined as the air temperature in the month when the NDVI or LAI reaches its
maximum for the year.
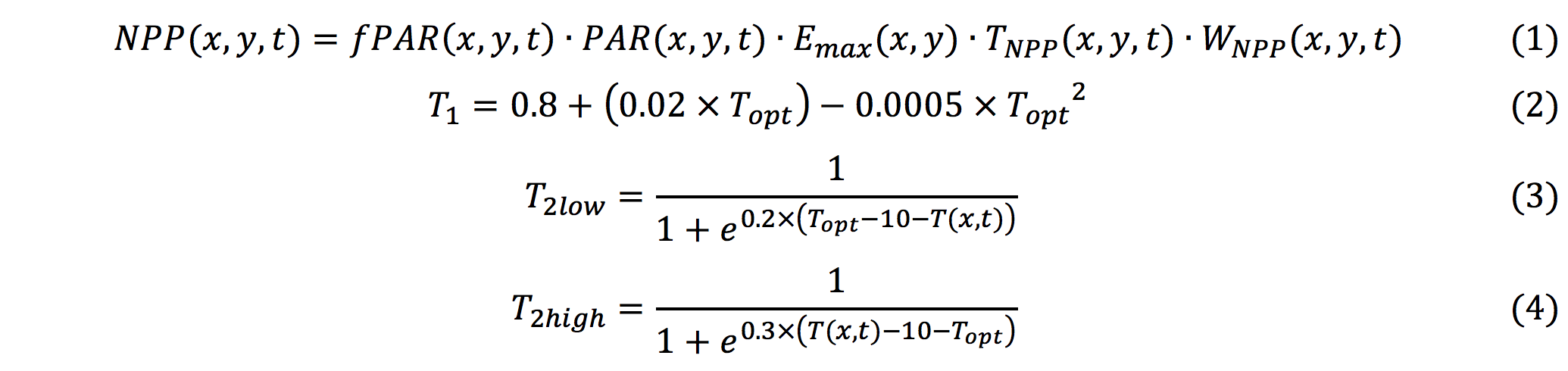
On
a monthly time step, NPP is allocated to leaves, roots and wood (Eq. 5), with a
default allocation ratio of 1:1:1. Each of these pools has a turnover time that
specifies the rate at which carbon moves to litter pools (surface fine litter,
soil fine litter, coarse woody debris). Carbon in the litterfall pool is either
transferred to the microbial and soil organic matter pools or decomposed during
the process (Fig. 1). Decomposition of dead pool (e.g. litter and soil organic
pools) releases carbon, i.e. heterotrophic respiration (Rh), as Eq. 6:

where
p is the number of pools, Ci is the carbon content of
pool i, ki is the pool-specific decay rate constant, Wresp and Tresp are the effect of soil
moisture and temperature on decomposition, and Dε is microbial carbon decomposition efficiency. The
effect of temperature on soil carbon fluxes (Tresp) is treated uniformly as an exponential (Q10)
response:

where Q10 is the
multiplicative increase in soil biological activity for a 10 ºC rise in soil
temperature and T(x, t) is monthly
averaged air temperature.
NEP
is computed as:

We
assumed a carbon use efficiency of 0.5 such that gross primary productivity
(GPP) is 2×NPP. Correspondingly, total ecosystem respiration (RECO) would
become the sum of NPP and Rh, and net ecosystem exchange (NEE) is equal to RECO
– GPP. The data used as input to the model are listed in section 4.
For 3-hourly simulation, we used the
North American Regional Reanlaysis (NARR) 3-hourly (UTC) air temperature (Tair) and downward shortwave
radiation (DWSW) to
further downscale monthly carbon fluxes. Here, we distributed monthly estimates
to 3hourly temporal scale with a simple assumption of dependence on light for
GPP and temperature for RECO (Olsen and Randerson
2004; Fisher et al. 2016).

where

5.2 Full parameter sets for generating L1 data
Table 2.
Perturbed parameter sets used to generate CASA ensemble Level-1 product.
#Para |
1 |
2 |
3 |
4 |
5 |
6 |
7 |
8 |
9 |
10 |
11 |
12 |
ΔTopt |
0 |
-2 |
2 |
0 |
-2 |
2 |
0 |
-2 |
2 |
0 |
-2 |
2 |
Emax |
0.25 |
0.25 |
0.25 |
0.5 |
0.5 |
0.5 |
0.75 |
0.75 |
0.75 |
1 |
1 |
1 |
Q10 |
1.4 |
1.4 |
1.4 |
1.4 |
1.4 |
1.4 |
1.4 |
1.4 |
1.4 |
1.4 |
1.4 |
1.4 |
#Para |
13 |
14 |
15 |
16 |
17 |
18 |
19 |
20 |
21 |
22 |
23 |
24 |
ΔTopt |
0 |
-2 |
2 |
0 |
-2 |
2 |
0 |
-2 |
2 |
0 |
-2 |
2 |
Emax |
0.25 |
0.25 |
0.25 |
0.5 |
0.5 |
0.5 |
0.75 |
0.75 |
0.75 |
1 |
1 |
1 |
Q10 |
1.2 |
1.2 |
1.2 |
1.2 |
1.2 |
1.2 |
1.2 |
1.2 |
1.2 |
1.2 |
1.2 |
1.2 |
#Para |
25 |
26 |
27 |
28 |
29 |
30 |
31 |
32 |
33 |
34 |
35 |
36 |
ΔTopt |
0 |
-2 |
2 |
0 |
-2 |
2 |
0 |
-2 |
2 |
0 |
-2 |
2 |
Emax |
0.25 |
0.25 |
0.25 |
0.5 |
0.5 |
0.5 |
0.75 |
0.75 |
0.75 |
1 |
1 |
1 |
Q10 |
1.6 |
1.6 |
1.6 |
1.6 |
1.6 |
1.6 |
1.6 |
1.6 |
1.6 |
1.6 |
1.6 |
1.6 |
37 |
38 |
39 |
40 |
41 |
42 |
43 |
44 |
45 |
(Para
37 – 45 for cropland only) |
|||
ΔTopt |
0 |
-2 |
2 |
0 |
-2 |
2 |
0 |
-2 |
2 |
|||
Emax |
1.25 |
1.25 |
1.25 |
1.25 |
1.25 |
1.25 |
1.25 |
1.25 |
1.25 |
|||
Q10 |
1.4 |
1.4 |
1.4 |
1.2 |
1.2 |
1.2 |
1.6 |
1.6 |
1.6 |
|||
Note: ΔTopt is the adjustment
of optimal temperature. |
5.3 Pruned parameter sets for generating L2 data
In order to further constrain Emax for each biome type, we use carbon flux
measurements during the growing seasons from AmeriFlux and FLUXNET datasets
(listed sites and corresponding years in Table S2) to infer the appropriate
biome-specific range of Emax
according to the light use efficiency model in CASA (Eq. 13). As flux sites are
broadly distributed across space, we defined the growing season as months when
the NPP is higher than averaged NPP within each year.

NPPobs_in is the inferred NPP value from flux measurement, fPAR is derived from MOD15A2H at each
flux site, and PARobs is
the ground-measured at each site (for sites lacking PAR observation, we used
NLDAS-2 instead). NPP scalars (TNPP
and WNPP) are computed
using ground-measured precipitation and air temperature (for sites lacking
these observations, we used data sampled from PRISM at corresponding flux tower
locations).
Table 3. Statistics of Emax inferred from flux tower data for each biome type
to generate Level-2 data.
WSA |
CRO |
DBF |
ENF |
MF |
GRA |
CSH |
OSH |
|
Grow Seas Avg |
0.51 |
1.01 |
0.69 |
0.64 |
0.51 |
0.69 |
0.47 |
0.4 |
Grow Seas STD |
0.04 |
0.37 |
0.15 |
0.23 |
0.29 |
0.29 |
0.29 |
0.15 |
Emax Samples for full Uncert. [E1, E2, E3] |
[0.25, 0.50, 0.50] |
[0.75, 1.00, 1.25] |
[0.50, 0.75, 0.75] |
[0.50, 0.75, 0.75] |
[0.25, 0.50, 0.75] |
[0.50, 0.75, 1.00] |
[0.25, 0.50, 0.75] |
[0.25, 0.50, 0.50] |
Table 4. Perturbed
parameter sets with constrained PFT-specific Emax used to generate
CASA ensemble Level-2 product.
#Para |
1 |
2 |
3 |
4 |
5 |
6 |
7 |
8 |
9 |
ΔTopt |
0 |
-2 |
2 |
0 |
-2 |
2 |
0 |
-2 |
2 |
Emax |
E1 |
E1 |
E1 |
E1 |
E1 |
E1 |
E1 |
E1 |
E1 |
Q10 |
1.4 |
1.4 |
1.4 |
1.2 |
1.2 |
1.2 |
1.6 |
1.6 |
1.6 |
#Para |
10 |
11 |
12 |
13 |
14 |
15 |
16 |
17 |
18 |
ΔTopt |
0 |
-2 |
2 |
0 |
-2 |
2 |
0 |
-2 |
2 |
Emax |
E2 |
E2 |
E2 |
E2 |
E2 |
E2 |
E2 |
E2 |
E2 |
Q10 |
1.4 |
1.4 |
1.4 |
1.2 |
1.2 |
1.2 |
1.6 |
1.6 |
1.6 |
#Para |
19 |
20 |
21 |
22 |
23 |
24 |
25 |
26 |
27 |
ΔTopt |
0 |
-2 |
2 |
0 |
-2 |
2 |
0 |
-2 |
2 |
Emax |
E3 |
E3 |
E3 |
E3 |
E3 |
E3 |
E3 |
E3 |
E3 |
Q10 |
1.4 |
1.4 |
1.4 |
1.2 |
1.2 |
1.2 |
1.6 |
1.6 |
1.6 |
Note: ΔTopt is the adjustment of optimal
temperature. |
5.4 Ecoregional sampling of Level-2 ensemble for generating Level-2B data
In addition to the Level-2 ensemble product, we added Level-2B to the data set which is the random sampling of Level-2 ensemble (27 members) based on the ecoregion maps. The Level-2B file, entitled with “CASA_L2B_Ensemble**”, has 10 members that randomly sampled L2 ensemble member (i.e., parameter set) for each Level-3 ecoregion for both North America and CONUS. Considering the data volume, we included only GPP and NEE for the Level-2B data. More information about ecoregion maps can be found at https://www.epa.gov/eco-research/ecoregions. Levels 1-3 ecoregion maps are available for North America; levels 1-4 ecoregion maps are available for conterminous US. The supplement contains an R script and converted ecoregion files (netcdf files) in order for users to generate the random sample for the ecoregion maps at other levels or change the number of samples.
5.5 Driver Data
Dataset |
Spatial
resolution |
Time
resolution |
Reference |
|
(a)
Conterminous US |
||||
fPAR |
463.31 m |
8-day |
Myneni et al. (2015) |
|
Tree and herb covers |
250 m |
Yearly |
Dimiceli et al. (2015) |
|
Precipitation and Tair |
PRISM |
30 ″ |
Monthly |
PRISM Climate Group (2016) |
DWSW and DWLW1 |
NDLAS-2 Forcing |
0.125 ° |
Monthly |
LDAS (2016) |
DWSW1 and Tair |
NARR |
32 km |
3-hourly |
NCEP (2005) |
National
Forest Type |
250 m |
NA |
Ruefenacht et al. (2008) |
|
NAFD |
30 m |
NA |
Goward et al. (2012) |
|
MOD12Q1 IGBP |
463.31
m |
Yearly |
Friedl et al. (2010) |
|
Clay, silt, sand Fractions |
1000 m |
NA |
Miller and White (1998) |
|
fPAR |
1000 m |
8-day |
Myneni et al. (2002) |
|
Tree and herb covers |
MOD44B |
250 m |
Yearly |
Dimiceli et al. (2015) |
Precipitation, Tair, DWSW, and DWLW1 |
NARR |
32 km |
Monthly |
NCEP (2005) |
DWSW and Tair |
NARR |
32 km |
3-hourly |
NCEP (2005) |
Biome type |
250 m |
NA |
Ruefenacht et al. (2008) |
|
30 m |
NA |
Goward et al. (2012) |
||
MOD12Q1 IGBP |
463.31 m |
Yearly |
Friedl et al. (2010) |
|
Clay, Silt, Sand Fractions |
NACP MsTMIP Soil Map |
0.25 ° |
NA |
Liu et al. (2014) |
1. DWSW and DWLW are downward shortwave and longwave radiation,
respectively. |
5.6 Guide of
using R script for temporal downscaling
We provide the temporal downscaling codes written in R
to enable users to estimate 3-hourly fluxes from monthly flux data. This script
performs a temporal downscaling of monthly carbon flux estimates from a CASA
model ensemble for two spatial domains, conterminous United States and North
America. The R script uses three packages, including ncdf4, rgdal, and raster. One the users’ end,
1) users need to prepare the time series of 3-hourly
NARR air temperature (in degree Celsius) and downward shortwave radiation for
each month, separately, and change the path (NARRPath) in the script. We
provide the 3-hoourly NARR examples for 2016-2018 with the R script;
2) users need to set the working dictionary to the
path saved monthly ensemble (MonthlyEnsemblePath), and put the reference maps
(NA_grid.tif and CONUS_grid.tif) into the working folder;
3) users can select the year (save3hrYear), month
(save3hrMonth) and parameter set (save3hrParaSet, default is all 27 parameter
sets) for temporal downscaling;
4) users can choose to save the 3-hourly outputs
(Save3hrGPP, Save3hrRECO, Save3hrNEE).
Questions on how to prepare the 3-hourly NARR data or
using this script can be forwarded to YuZhou2@clarku.edu (or CWilliams@clarku.edu)
5.7 Guide of using R script for random ecoregional sampling (Level-2B)
We provide a R script of random ecoregional sampling to generate the Level-2 ensemble at users’ end for two spatial domains, conterminous United States and North America. The R script uses three packages, including ncdf4, rgdal, and raster. On the users’ end,
1) users need to determine which ecoregional level to work with by define "EcoregionLevel". Levels 1-3 are available for North America; levels 1-4 are available for conterminous US. Here we have converted shapefiles of different levels from United States Environmental Protection Agency (https://www.epa.gov/eco-research/ecoregions) to the netcdf files that can be directly used in this script;
2) users need to define the spatial domain of the random ecoregional sampling: conterminous United States (CONUS) or North America (NA);
3) users need to set the path of ecoregion files (e.g., if users are working with level-3 ecoregions for conterminous United States, the ecoregion file is CONUS_Eco_Level3_CASAgrid.nc4);
4) users can define the number of Level-2B sampling by change "L2BMembers";
5) users need to set the path of Level-2 files by change "L2Path";
6) users can select the year(s) ("SampleYear") for sampling;
7) users can select the carbon flux(es) ("CFluxes") to be sampled;
8) If users would like to use the previous random samples for another sampling of a same spatial domain, please change "Saved_EcoregionRandSamp" to 1 and move the file "EcoregionRandSamp_**.txt" to the output path. This file should be found in the output path when "Saved_EcoregionRandSamp" is set to 0.
9) users can set the output path ("outputPath").
Questions on using this script can be forwarded to YuZhou2@clarku.edu (or cwilliams@clarku.edu)
These data are
available through the ACT-America Model Data Repository hosted at the Oak Ridge National Laboratory.
Data Access Link: ftp://evs2ftp.ornl.gov/Prior_Fluxes/Ecosystem_Fluxes/CASA_Ensemble/
Contact for
Data Access Information:
E-mail:
weiy@ornl.gov
For
the L1 product, we perturbed the most sensitive parameters with the full 36 member
suite of parameters (Table 3). This level of the product is not available
online, please contact CWilliams@clarku.edu if you would
like to use our L1 product.
Acknowledgement
This work was primarily funded by the Atmospheric Carbon and Transport (ACT) - America project, a NASA Earth Venture Suborbital 2 project supported by NASA’s Earth Science Division. Funding for this work came from the NASA ACT-America Project under award #NNX16AN17G and NNX15AG76G. This work used eddy covariance data acquired and shared by the FLUXNET community, including AmeriFlux and Fluxnet-Canada. Funding for AmeriFlux data resources was provided by the U.S. Department of Energy’s Office of Science. CarbonTracker (CT2017) results provided by NOAA ESRL, Boulder, Colorado, USA from the website at http://carbontracker.noaa.gov. Funding for the Multi-scale synthesis and Terrestrial Model Intercomparison Project (MsTMIP; https://nacp.ornl.gov/MsTMIP.shtml) activity was provided through NASA ROSES Grant #NNX10AG01A. Data management support for preparing, documenting, and distributing model driver and output data was performed by the Modeling and Synthesis Thematic Data Center at Oak Ridge National Laboratory (ORNL; http://nacp.ornl.gov), with funding through NASA ROSES Grant #NNH10AN68
7.
References
Baker, I., Harper, A., da Rocha, H., Denning, A., Araújo, A., Borma, L., Freitas, H., Goulden, M., Manzi, A., & Miller, S. 2013. Surface ecophysiological behavior across vegetation and moisture gradients in tropical South America. Agricultural and Forest Meteorology, 182, 177-188
Baker,
I., Prihodko, L., Denning, A., Goulden, M., Miller, S., & Da Rocha, H.
2008. Seasonal drought stress in the Amazon: Reconciling models and
observations. Journal of Geophysical
Research: Biogeosciences, 113
Baker, J., & Griffis, T. 2017. AmeriFlux US-Ro2 Rosemount- C7, doi:10.17190/AMF/1418683
Belshe,
E., Schuur, E., Bolker, B., & Bracho, R. 2012. Incorporating spatial
heterogeneity created by permafrost thaw into a landscape carbon estimate. Journal of Geophysical Research: Biogeosciences,
117
Billesbach, D., Bradford, J., & Torn, M. 2016a. AmeriFlux US-AR1 ARM USDA UNL OSU Woodward Switchgrass 1, doi:10.17190/AMF/1246137
Billesbach, D., Bradford, J., & Torn, M. 2016b. AmeriFlux US-AR2 ARM USDA UNL OSU Woodward Switchgrass 2, doi:10.17190/AMF/1246138
Bowling, D. 2007. AmeriFlux US-Cop Corral Pocket, doi:10.17190/AMF/1246129
Brunsell, N. 2018a. AmeriFlux US-KFS Kansas Field Station, doi:10.17190/AMF/1246132
Brunsell, N. 2018b. AmeriFlux US-Kon Konza Prairie LTER (KNZ), doi:10.17190/AMF/1246068
Chen, J. 2003a. AmeriFlux US-Wi1 Intermediate hardwood (IHW), doi:10.17190/AMF/1246015
Chen, J. 2003b. AmeriFlux US-Wi2 Intermediate red pine (IRP), doi:10.17190/AMF/1246017
Chen, J. 2003c. AmeriFlux US-Wi6 Pine barrens #1 (PB1), doi:10.17190/AMF/1246021
Chen, J. 2004. AmeriFlux US-Wi5 Mixed young jack pine (MYJP), doi:10.17190/AMF/1246020
Chen, J. 2005a. AmeriFlux US-Wi7 Red pine clearcut (RPCC), doi:10.17190/AMF/1246022
Chen, J. 2005b. AmeriFlux US-Wi9 Young Jack pine (YJP), doi:10.17190/AMF/1246024
Cook, B.D., Davis, K.J., Wang, W., Desai, A., Berger, B.W., Teclaw, R.M., Martin, J.G., Bolstad, P.V., Bakwin, P.S., & Yi, C. 2004. Carbon exchange and venting anomalies in an upland deciduous forest in northern Wisconsin, USA. Agricultural and Forest Meteorology, 126, 271-295
Desai, A.R., Bolstad, P.V., Cook, B.D., Davis, K.J., & Carey, E.V. 2005. Comparing net ecosystem exchange of carbon dioxide between an old-growth and mature forest in the upper Midwest, USA. Agricultural and Forest Meteorology, 128, 33-55
Desai, A.R., Xu, K., Tian, H., Weishampel, P., Thom, J., Baumann, D., Andrews, A.E., Cook, B.D., King, J.Y., & Kolka, R. 2015. Landscape-level terrestrial methane flux observed from a very tall tower. Agricultural and Forest Meteorology, 201, 61-75
Dimiceli,
C., Carroll, M., Sohlberg, R., Kim, D.H., Kelly, M., & Townshend, J.R.G.
2015. MOD44B MODIS/Terra Vegetation Continuous Fields Yearly L3 Global 250m SIN
Grid V006. NASA EOSDIS Land Processes
DAAC. Available online: https://lpdaac.usgs.gov/dataset_discovery/modis/modis_products_table/mod44b_v006
(accessed on 26 July 2016)
Ewers, B., & Pendall, E. 2009. AmeriFlux US-Sta Saratoga, doi:10.17190/AMF/1246831
Fares, S. 2010. AmeriFlux US-Lin Lindcove Orange Orchard, doi:10.17190/AMF/1246830
Fischer, M.L., Billesbach, D.P., Berry, J.A., Riley, W.J., & Torn, M.S. 2007. Spatiotemporal variations in growing season exchanges of CO2, H2O, and sensible heat in agricultural fields of the Southern Great Plains. Earth interactions, 11, 1-21
Fisher, J.B., Sikka, M., Huntzinger, D.N., Schwalm, C., & Liu, J. 2016. 3-hourly temporal downscaling of monthly global terrestrial biosphere model net ecosystem exchange. Biogeosciences, 13, 4271-4277
Frank, J.M., Massman, W.J., Ewers, B.E., Huckaby, L.S., & Negrón, J.F. 2014. Ecosystem CO2/H2O fluxes are explained by hydraulically limited gas exchange during tree mortality from spruce bark beetles. Journal of Geophysical Research: Biogeosciences, 119, 1195-1215
Friedl, M.A., Sulla-Menashe, D., Tan, B., Schneider, A., Ramankutty, N., Sibley, A., & Huang, X. 2010. MODIS Collection 5 global land cover: Algorithm refinements and characterization of new datasets. Remote Sensing of Environment, 114, 168-182
Goldstein, A., Hultman, N., Fracheboud, J., Bauer, M., Panek, J., Xu, M., Qi, Y., Guenther, A., & Baugh, W. 2000. Effects of climate variability on the carbon dioxide, water, and sensible heat fluxes above a ponderosa pine plantation in the Sierra Nevada (CA). Agricultural and Forest Meteorology, 101, 113-129
Gough, C., Bohrer, G., & Curtis, P. 2018. AmeriFlux US-UMd UMBS Disturbance, doi:10.17190/AMF/1246134
Gough, C., Vogel, C., Schmid, H., Su, H.-B., & Curtis, P. 2008. Multi-year convergence of biometric and meteorological estimates of forest carbon storage. Agricultural and Forest Meteorology, 148, 158-170
Goward, S.N., Huang, C., Masek, J.G., Cohen, W.B., Moisen, G.G., & Schleeweis, K. 2012. NACP North American Forest Dynamics Project: Forest Disturbance and Regrowth Data. ORNL DAAC, Oak Ridge, Tennessee, USA. http://dx.doi.org/10.3334/ORNLDAAC/1077
Hadley, J. 2018. AmeriFlux US-LPH Little Prospect Hill, doi:10.17190/AMF/1246072
Hatala, J.A., Detto, M., Sonnentag, O., Deverel, S.J., Verfaillie, J., & Baldocchi, D.D. 2012. Greenhouse gas (CO2, CH4, H2O) fluxes from drained and flooded agricultural peatlands in the Sacramento-San Joaquin Delta. Agriculture, ecosystems & environment, 150, 1-18
Hollinger, D., Goltz, S., Davidson, E., Lee, J., Tu, K., & Valentine, H. 1999. Seasonal patterns and environmental control of carbon dioxide and water vapour exchange in an ecotonal boreal forest. Global Change Biology, 5, 891-902
Huntzinger, D., Schwalm, C., Michalak, A., Schaefer, K., King, A., Wei, Y., Jacobson, A., Liu, S., Cook, R., & Post, W. 2013. The north american carbon program multi-scale synthesis and terrestrial model intercomparison project–part 1: Overview and experimental design. Geoscientific Model Development, 6, 2121-2133
Huntzinger, D., Schwalm, C., Wei, Y., Cook, R., Michalak, A., Schaefer, K., Jacobson, A., Arain, M., Ciais, P., Fisher, J., Hayes, D.J., Huang, M., Huang, S., Ito, A., Jain, A.K., et al. 2016. NACP MsTMIP: Global 0.5-deg Terrestrial Biosphere Model Outputs (version 1) in Standard Format. ORNL DAAC, Oak Ridge, Tennessee, USA. https://doi.org/10.3334/ORNLDAAC/1225.
Kurc, S. 2018. AmeriFlux US-SRC Santa Rita Creosote, doi:10.17190/AMF/1246127
Law, B. 2018. AmeriFlux US-MRf Mary's River (Fir) site, doi:10.17190/AMF/1246049
LDAS 2016. NLDAS-2 Forcing Dataset, Land Data Assimilation Systems, at https://ldas.gsfc.nasa.gov/nldas/NLDAS2forcing.php (accessed on June 10, 2016)
Litvak, M. 2018a. AmeriFlux US-Mpj Mountainair Pinyon-Juniper Woodland, doi:10.17190/AMF/1246123
Litvak, M. 2018b. AmeriFlux US-Wjs Willard Juniper Savannah, doi:10.17190/AMF/1246120
Liu, S., Wei, Y., Post, W., Cook, R., Schaefer, K., & Thornton, M. 2014. NACP MsTMIP: Unified North American soil map. Available online: http://dx.doi.org/10.3334/ORNLDAAC/1242, Oak Ridge National Laboratory Distributed Active Archive Center, Oak Ridge, Tennessee, USA (accessed on 27 Jan 2017). In
Massman, B. 2006. AmeriFlux US-GBT GLEES Brooklyn Tower, doi:10.17190/AMF/1375200
Matamala, R. 2018. AmeriFlux US-IB2 Fermi National Accelerator Laboratory- Batavia (Prairie site), doi:10.17190/AMF/1246066
Miller, D.A., & White, R.A. 1998. A conterminous United States multilayer soil characteristics dataset for regional climate and hydrology modeling. [Available at http://EarthInteractions.org]. Earth interactions, 2, 1-26
Monson, R., Turnipseed, A., Sparks, J., Harley, P., Scott‐Denton, L., Sparks, K., & Huxman, T. 2002. Carbon sequestration in a high‐elevation, subalpine forest. Global Change Biology, 8, 459-478
Myneni,
R., Knyazikhin, Y., & Park, T. 2015. MCD15A2H MODIS/Terra+Aqua Leaf Area
Index/FPAR 8-day L4 Global 500m SIN Grid V006. NASA EOSDIS Land Processes DAAC. Available online: https://lpdaac.usgs.gov/dataset_discovery/modis/modis_products_table/mcd15a2h_v006
(accessed on 28 July 2016)
Myneni, R.B., Hoffman, S., Knyazikhin, Y., Privette, J., Glassy, J., Tian, Y., Wang, Y., Song, X., Zhang, Y., & Smith, G. 2002. Global products of vegetation leaf area and fraction absorbed PAR from year one of MODIS data. Remote Sensing of Environment, 83, 214-231
Nakai, T., Kim, Y., Busey, R.C., Suzuki, R., Nagai, S., Kobayashi, H., Park, H., Sugiura, K., & Ito, A. 2013. Characteristics of evapotranspiration from a permafrost black spruce forest in interior Alaska. Polar Science, 7, 136-148
NCEP, N.W.S.N.U.S.D.o.C. 2005. National Centers for Environmental Prediction (NCEP) North American Regional Reanalysis (NARR). In. Boulder, CO: Research Data Archive at the National Center for Atmospheric Research, Computational and Information Systems Laboratory
Noormets, A., McNulty, S.G., DeForest, J.L., Sun, G., Li, Q., & Chen, J. 2008. Drought during canopy development has lasting effect on annual carbon balance in a deciduous temperate forest. New Phytologist, 179, 818-828
Olsen,
S.C., & Randerson, J.T. 2004. Differences between surface and column
atmospheric CO2 and implications for carbon cycle research. Journal of Geophysical Research:
Atmospheres, 109
Peters, W., Jacobson, A.R., Sweeney, C., Andrews, A.E., Conway, T.J., Masarie, K., Miller, J.B., Bruhwiler, L.M., Pétron, G., & Hirsch, A.I. 2007. An atmospheric perspective on North American carbon dioxide exchange: CarbonTracker. Proceedings of the National Academy of Sciences, 104, 18925-18930
Potter, C.S., Randerson, J.T., Field, C.B., Matson, P.A., Vitousek, P.M., Mooney, H.A., & Klooster, S.A. 1993. Terrestrial ecosystem production: a process model based on global satellite and surface data. Global Biogeochemical Cycles, 7, 811-841
Powell, T.L., Bracho, R., Li, J., Dore, S., Hinkle, C.R., & Drake, B.G. 2006. Environmental controls over net ecosystem carbon exchange of scrub oak in central Florida. Agricultural and Forest Meteorology, 141, 19-34
PRISM Climate Group 2016. PRISM Gridded Climate Data, Oregon State University, http://prism.oregonstate.edu (accessed on 20 July 2016). In
Randerson, J.T., Thompson, M.V., Malmstrom, C.M., Field, C.B., & Fung, I.Y. 1996. Substrate limitations for heterotrophs: Implications for models that estimate the seasonal cycle of atmospheric CO2. Global Biogeochemical Cycles, 10, 585-602
Ruefenacht, B., Finco, M., Nelson, M., Czaplewski, R., Helmer, E., Blackard, J., Holden, G., Lister, A., Salajanu, D., & Weyermann, D. 2008. Conterminous US and Alaska forest type mapping using forest inventory and analysis data. Photogrammetric Engineering & Remote Sensing, 74, 1379-1388
Ruehr, N.K., Martin, J.G., & Law, B.E. 2012. Effects of water availability on carbon and water exchange in a young ponderosa pine forest: Above-and belowground responses. Agricultural and Forest Meteorology, 164, 136-148
Schmid, H.P., Grimmond, C.S.B., Cropley, F., Offerle, B., & Su, H.-B. 2000. Measurements of CO2 and energy fluxes over a mixed hardwood forest in the mid-western United States. Agricultural and Forest Meteorology, 103, 357-374
Scott, R.L., Biederman, J.A., Hamerlynck, E.P., & Barron‐Gafford, G.A. 2015. The carbon balance pivot point of southwestern US semiarid ecosystems: Insights from the 21st century drought. Journal of Geophysical Research: Biogeosciences, 120, 2612-2624
Scott,
R.L., Hamerlynck, E.P., Jenerette, G.D., Moran, M.S., & Barron‐Gafford,
G.A. 2010. Carbon dioxide exchange in a semidesert grassland through drought‐induced
vegetation change. Journal of Geophysical
Research: Biogeosciences, 115
Scott,
R.L., Jenerette, G.D., Potts, D.L., & Huxman, T.E. 2009. Effects of seasonal
drought on net carbon dioxide exchange from a woody‐plant‐encroached
semiarid grassland. Journal of
Geophysical Research: Biogeosciences, 114
Thomas,
C.K., Law, B.E., Irvine, J., Martin, J.G., Pettijohn, J.C., & Davis, K.J.
2009. Seasonal hydrology explains interannual and seasonal variation in carbon
and water exchange in a semiarid mature ponderosa pine forest in central
Oregon. Journal of Geophysical Research:
Biogeosciences, 114
Torn, M. 2006a. AmeriFlux US-ARb ARM Southern Great Plains burn site- Lamont, doi:10.17190/AMF/1246025
Torn, M. 2006b. AmeriFlux US-ARc ARM Southern Great Plains control site- Lamont, doi:10.17190/AMF/1246026
Urbanski,
S., Barford, C., Wofsy, S., Kucharik, C., Pyle, E., Budney, J., McKain, K.,
Fitzjarrald, D., Czikowsky, M., & Munger, J. 2007. Factors controlling CO2
exchange on timescales from hourly to decadal at Harvard Forest. Journal of Geophysical Research:
Biogeosciences, 112
Verma, S.B., Dobermann, A., Cassman, K.G., Walters, D.T., Knops, J.M., Arkebauer, T.J., Suyker, A.E., Burba, G.G., Amos, B., & Yang, H. 2005. Annual carbon dioxide exchange in irrigated and rainfed maize-based agroecosystems. Agricultural and Forest Meteorology, 131, 77-96
Vickers, D., Thomas, C., & Law, B.E. 2009. Random and systematic CO2 flux sampling errors for tower measurements over forests in the convective boundary layer. Agricultural and Forest Meteorology, 149, 73-83
Wilson, T., & Meyers, T. 2007. Determining vegetation indices from solar and photosynthetically active radiation fluxes. Agricultural and Forest Meteorology, 144, 160-179